How Machine Learning is Revolutionizing Mobile App Performance
Author: Admin
September 20, 2024
machine learning in mobile apps app performance optimization user retention strategies predictive analytics intelligent error detection personalized user experience A/B testing. 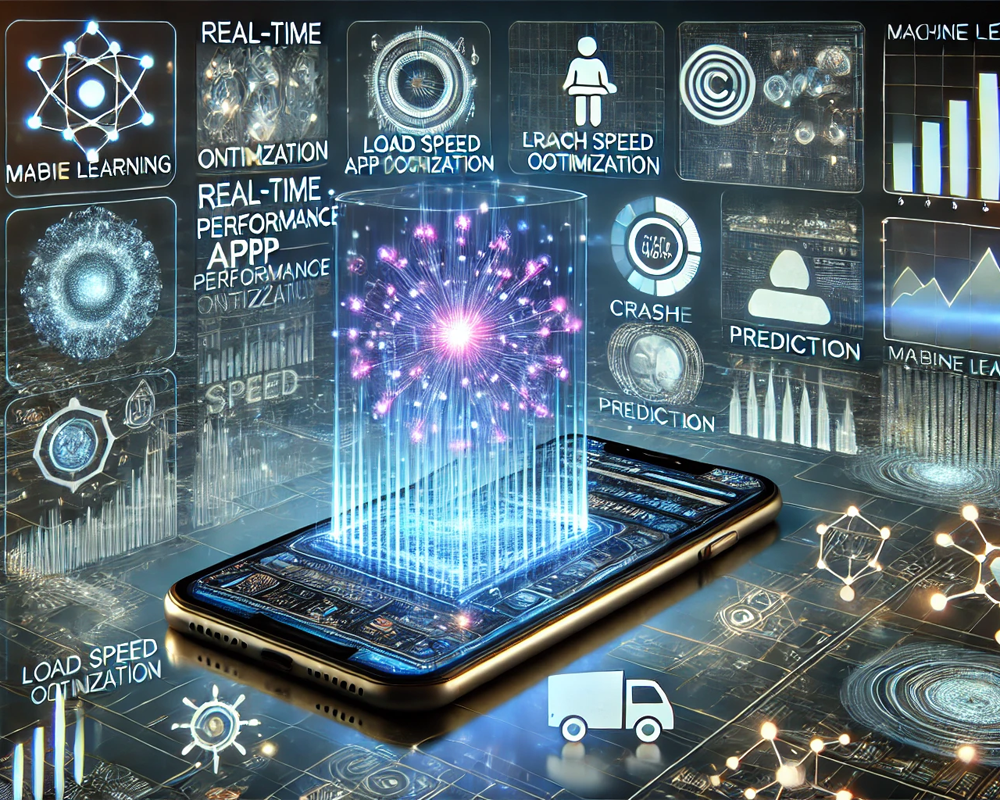
In the ever-evolving world of mobile app development, Machine Learning (ML) is emerging as a game-changer. By integrating ML algorithms into mobile apps, developers are not only enhancing app performance but also improving user retention. This revolutionary technology leverages data-driven insights to optimize various aspects of app functionality, ensuring a smoother, more personalized experience for users. In this article, we delve into how machine learning is transforming mobile app performance and boosting user engagement.
Personalized User Experiences
Machine learning excels in analyzing user data to deliver personalized experiences. By understanding individual preferences and behaviors, ML algorithms tailor app interactions to suit each user’s needs.
- Content Recommendations: Apps like Netflix and Spotify use ML to analyze users’ past interactions and predict future preferences. This results in personalized content recommendations that keep users engaged and coming back for more.
- Customized Interfaces: ML can adjust app interfaces based on user behavior. For instance, if a user frequently accesses certain features, these can be highlighted or made more accessible, enhancing the overall usability of the app.
Predictive Performance Optimization
Machine learning algorithms can predict app performance issues before they impact users. By analyzing historical data and identifying patterns, ML helps in proactive performance management.
- Load Time Predictions: ML models can forecast high-traffic periods and adjust server resources accordingly. This prevents slow load times during peak usage and ensures a smooth experience for all users.
- Resource Allocation: ML can optimize how resources are allocated within an app. For example, it can manage memory usage and processing power more efficiently, reducing lag and enhancing performance.
Intelligent Error Detection and Resolution
Machine learning improves app reliability by identifying and resolving errors more effectively.
- Anomaly Detection: ML algorithms monitor app behavior in real-time to detect anomalies that may indicate bugs or performance issues. By quickly identifying these anomalies, developers can address issues before they affect users.
- Automated Bug Fixes: Advanced ML systems can not only detect errors but also suggest or implement automated fixes. This reduces downtime and ensures that users experience fewer disruptions.
Enhanced User Retention Strategies
User retention is a critical metric for app success, and machine learning plays a key role in optimizing it.
- Behavioral Analytics: ML analyzes user behavior to identify patterns and trends. By understanding what keeps users engaged, apps can implement features or strategies that enhance user retention.
- Churn Prediction: ML models can predict which users are likely to abandon the app based on their behavior. Developers can then take proactive measures, such as targeted notifications or personalized offers, to retain these users.
Dynamic Personalization Through Real-Time Data
Machine learning enables real-time personalization, adapting the app experience as users interact with it.
- Adaptive Content Delivery: ML algorithms analyze user interactions on the fly and adjust content delivery accordingly. This ensures that users always see the most relevant content based on their current activity.
- Context-Aware Features: Apps can use ML to adapt features based on the user’s context, such as location or time of day. For instance, a navigation app might provide real-time traffic updates and route suggestions based on the user’s current location.
Improved User Support Through Chatbots
Machine learning enhances user support by powering intelligent chatbots that provide instant assistance.
- Natural Language Processing (NLP): ML-driven NLP enables chatbots to understand and respond to user queries more effectively. These chatbots can handle a wide range of questions and issues, providing immediate support and improving user satisfaction.
- Learning from Interactions: Chatbots powered by ML learn from each interaction, improving their responses over time. This continuous learning process helps in delivering more accurate and helpful support.
Efficient A/B Testing and Feature Optimization
Machine learning streamlines the A/B testing process, allowing developers to test and optimize app features more efficiently.
- Automated A/B Testing: ML can automate the process of running A/B tests by quickly analyzing user responses to different features or designs. This helps in identifying the most effective options without manual intervention.
- Feature Prioritization: By analyzing user feedback and interaction data, ML algorithms can prioritize features that have the highest impact on user satisfaction and engagement.
Conclusion
Machine learning is revolutionizing mobile app development by optimizing performance and enhancing user retention. From delivering personalized experiences and predicting performance issues to improving user support and streamlining feature testing, ML algorithms are at the forefront of creating more efficient, engaging, and user-friendly mobile apps. As technology continues to advance, the role of machine learning in mobile app development will become increasingly vital, driving innovation and setting new standards for app performance.